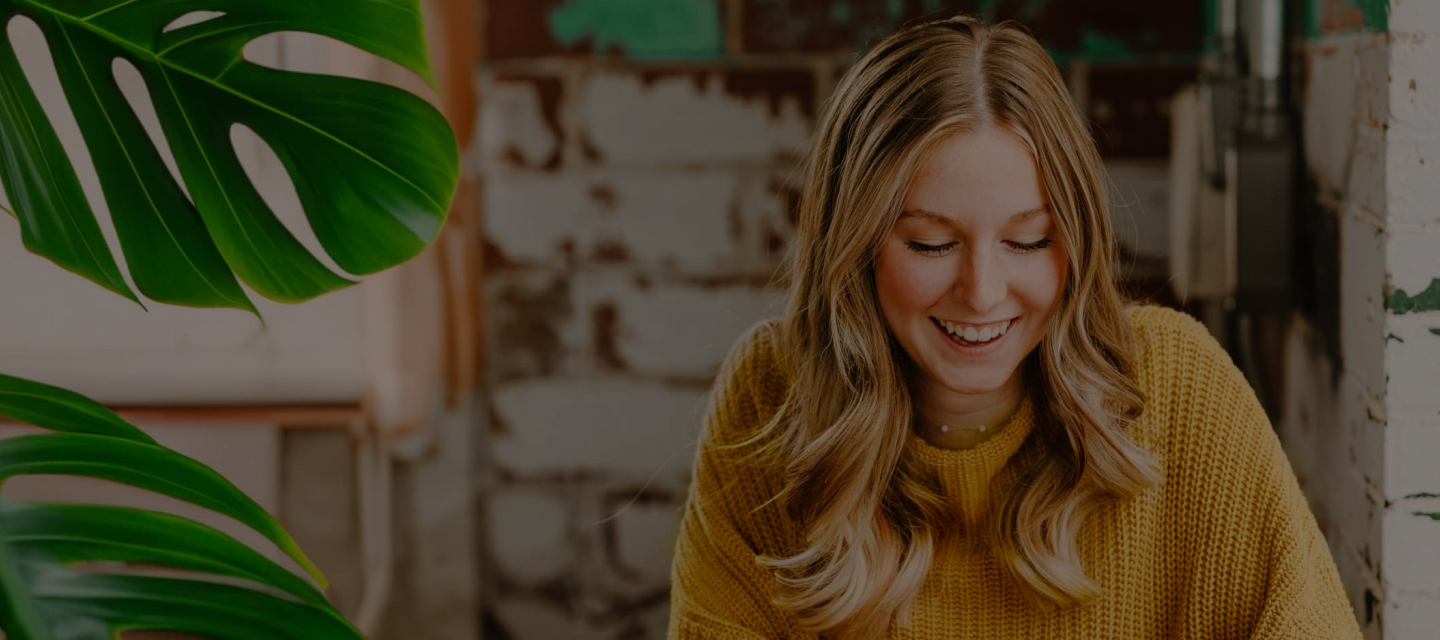
Input Data Validation
Ensuring Quality: Comprehensive Input Data Validation QA for AI Systems
Talk to our ExpertsFor any AI System to perform successfully, the input data has to be free of any errors. Thus, the initial step for AI testing is checking the data. So, when it comes to the testing of AI system at Tx, our experts ensure the input data of an AI system is well scrubbed, cleaned and validated. We check the input data to be free of any kind of human bias or any kind of variation.
Input Data Validation Challenges and Solutions
- Ensuring all data points follow the same standards and eliminating the conflicting details
- Obtaining accurate labels reflecting real-world conditions
- Protecting data from unauthorized access and potential corruption
- Implementing data governance frameworks that address data quality issues effectively
- Ensuring All Data Points Follow the Same Standards and Eliminating Conflicting Details
- Obtaining Accurate Labels Reflecting Real-World Conditions
- Protecting Data from Unauthorized Access and Potential Corruption
- Implementing Data Governance Frameworks that Address Data Quality Issues Effectively
Benefits of Validated Data
Get In Touch- Ensure accurate results
- Ensure data compatibility from various resources
- Save time down the line
- Avoid legal risks
Speak to an expert
In your line of work, we know every minute matters.
How do we Do it?
Collect Requirements
Covering the basics, including schema, quality attributes, freshness, access, ownership, and more.
Build the Pipeline
Considering components beyond data quality, including how pipeline needs to be handled, idempotency, and data optimization.
Sample the data, smoke test, data diff
Run the pipeline on a small subset of data to identify any easy spots, inconsistencies, or errors.
Write and implement data validation tests
Ensuring schema continuity as a part of a data contract is another common data validation test.
Continuously Improve and Deploy
Checking back in with data consumers to gauge satisfaction levels and surface any additional requirements.
Our Input Data Validation Differentiators
Applying rigorous data quality checks that encompass accuracy, completeness, consistency, and timeliness
Leveraging cutting-edge technologies, we identify and address anomalies in data sets
Eliminate corrupt or irrelevant data, enhancing the efficiency and accuracy of your AI applications.
Adhere to relevant data protection regulations, reducing legal risks and building trust with your stakeholders
Continuous data validation support to adapt to new data and evolving AI models