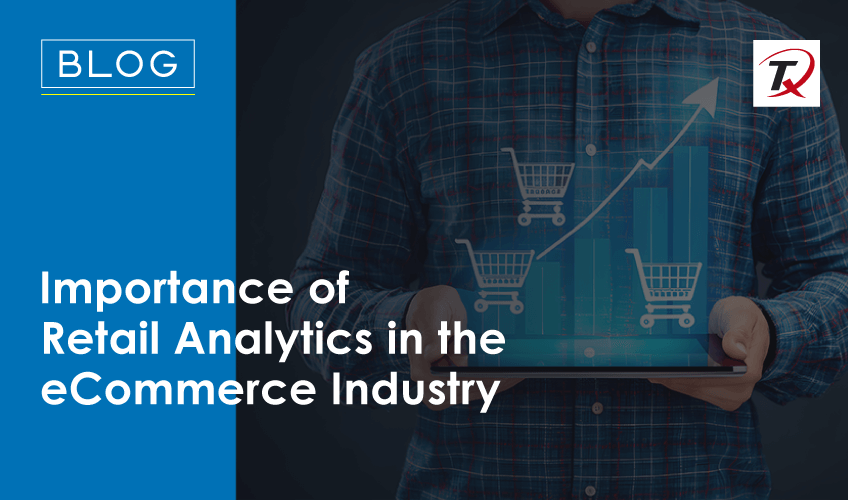
The retail industry has rapidly transformed in recent years due to technological advancements like AI/ML, RPA, etc. Evolving tech innovations, changing consumer demands, and global competition force retailers to remain competitive for business success. Especially in eCommerce retail, business owners must focus on technology, CX, and security. They must use their years of experience to decide which item to enlist at what location and the target audience, analyze geographical market conditions, and determine what type of marketing campaign would draw more business. Although retailers often listen to their instincts, it is no longer impactful in the digital business age, especially in the eCommerce industry, where profit margins are very narrow. Consumers have numerous options, and market conditions vary based on demand. This is where retail analytics comes into play.
Retail data analytics software can help eCommerce retailers make decisions and drive growth. According to McKinsey, businesses that integrate modern AI and deep learning techniques (part of advanced analytics) can experience enhanced performance by 30% to 128%, depending upon the quality of data available and specific applications. Retail analytics allows retailers to understand consumer behavior, optimize inventory, personalize marketing efforts, and enhance CX, vital for upscaling eCommerce operations.
Retail Analytics and its Importance for eCommerce Business
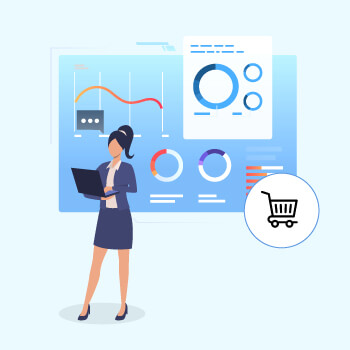
Retail analytics is the process of collecting, analyzing, and interpreting data derived from various sources and putting it through the analytics engines. The data sources include cash registers, POS devices, ERP, inventory and supply chain data, pricing, discounts, financial ledgers, etc. It uses software to analyze data collected from all these sources and deliver insights about consumer behaviour, shopping trends, inventory counts, etc., to the retailers. It can also improve the decision-making process about inventory, marketing campaigns, pricing, eCommerce store operations, merchandising, etc., by implementing predictive algorithms against internal and external repositories.
Retail analytics also helps measure user loyalty levels, predict demand forecasts, identify buying patterns, and optimize inventory layout. Thus, retailers can offer personalized discounts on their eCommerce platforms, which could result in higher average cart sizes and frequent site visits. Following are some of the factors that show why retail analytics is important for eCommerce businesses:
• eCommerce retailers can track and analyze customer interactions across various digital platforms. It will identify patterns and trends in consumer behavior, such as peak shopping hours, customer preferences, and popular products being sold. Retailers can use this data to tailor marketing efforts and product offerings to meet customer needs better.
• Retail analytics insights enable eCommerce businesses to create effective marketing campaigns. For example, retailers can tailor targeted cross-selling strategies by analyzing the frequently viewed product. Also, by analyzing the user journey, they can optimize advertising costs by focusing on effective touchpoints and channels.
• It helps in streamlining operations by monitoring inventory levels and sales data. Retailers can manage demand forecasts and stock levels to reduce holding costs and prevent out-of-stock situations.
• It helps improve sales growth by better understanding consumer and market needs. Analytics allow businesses to make data-driven decisions to facilitate sales improvement.
Types of Retail Data Analytics
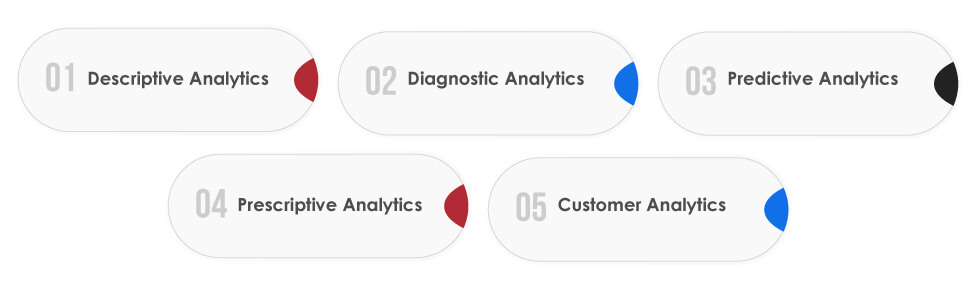
Retail data analytics is divided into five main types, each serving a specific purpose and providing insights into various retail operations. Having an understanding of these types would allow eCommerce businesses to utilize data for decision-making and create strategies:
Descriptive Analytics:
Descriptive Analytics is the foundation of retail data analytics. It includes summarizing historical data in an understandable format so that retailers can analyze past events. It enables businesses to track metrics like average transaction size, total sales, and yearly growth. This builds a solid foundation for understanding patterns and trends that affect business performance.
Diagnostic Analytics:
Diagnostic analytics allows eCommerce retailers to identify and analyze issues responsible for low performance. It combines data from multiple sources and uses techniques like drill-down, data discovery, cause-and-effect analysis, and correlation. For example, if sales drop in a particular quarter, diagnostic analytics will help reveal the cause, which could be a stock outage, pricing error, etc.
Predictive Analytics:
It helps retailers predict future events based on variables such as weather, supply chain disruptions, global market trends, economic trends, and competitive pressure. Predictive analytics is also known as what-if analysis. For instance, what action should be taken if a product runs out of stock due to certain actions like discount offers, short-duration rise in demand, etc.?
Prescriptive Analytics:
Prescriptive analytics involves utilizing AI and Big Data to analyze predictive analytics outcomes and create recommended actions, such as increasing customer satisfaction, improving CX, or maximizing profits. This type of analytics is necessary in decision-making processes.
Customer Analytics:
It is a subset of retail analytics that focuses on collecting and analyzing data related to customer behavior, demographics, and shopping needs. eCommerce businesses can use this data to tailor their marketing strategies, improve customer engagement, and optimize retention strategies.
5 eCommerce and Retail Analytics Use Cases
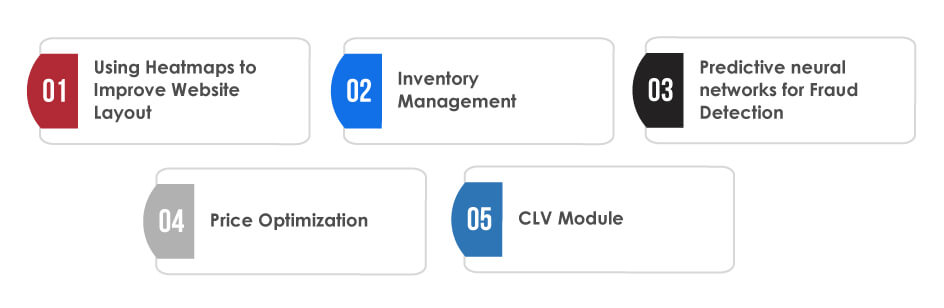
Retail analytics applications can transform eCommerce operations, making them more customer-centric and data-driven. Following are some of the compelling retail analytics use cases to enhance eCommerce operations:
Using Heatmaps to Improve Website Layout:
One of the coolest features of business intelligence tools is Heatmaps. These maps provide a visual representation of the website UI or store layout. The darker elements represent the higher traffic, or the spaces users clicked the most. The lighter elements represent lesser traffic or spaces where users clicked very little. Heatmaps can enable eCommerce retailers to analyze customer behaviors and make better business decisions. For instance, eCommerce retailers can analyze what is interactive to their customers and why and where the bounce rate is high. What is working for the business, and what is not? eCommerce retailers can use this data to create a better business strategy.
Inventory Management:
Effective inventory management is crucial for handling supply chain efficiency and reducing costs. eCommerce businesses can predict product demand using retail analytics, allowing them to optimize inventory levels. Retail analytics helps predict product demand, allowing businesses to optimize their inventory levels and prevent overstocking and understocking. This, in turn, ensures popular products are always available in eCommerce stores.
Predictive neural networks for Fraud Detection:
Deep neural networks help in fraud detection activity to avoid bad user experience. These are highly efficient in identifying fraudulent behavior, flagging it, and predicting any type of fraud. Incidents like return or delivery scams, consumer rights abuse, and credit risks can damage the retailer’s reputation. Predictive neural networks in retail analytics can safeguard against such frauds.
Price Optimization:
Retail analytics enable retailers to optimize their pricing strategies. It uses competitors’ data to compare prices, consumer demand, and cost dynamics, enabling eCommerce businesses to set prices that maximize profits and remain appealing to customers. Dynamic pricing models can adjust prices in real time depending on demand and inventory levels, which is crucial during sales or promotional periods.
CLV Module:
One of the major aspects of retail analytics is customer lifetime value (CLV) analytics. eCommerce retailers spend a lot on customer retention and acquisition activities. The CLV modules analyze the user behavior from their first transaction data to the most recent one to provide a comprehensive overview of the CX and what areas of the website need optimization. It also helps enhance the usability and customer satisfaction index, improving loyalty and business enhancement.
Retail Analytics Best Practices for eCommerce Business
Retail analytics can significantly improve eCommerce platforms’ decision-making and operational efficiency when implemented effectively. Following are some of the key best practices to ensure retail analytics can deliver the best outcomes for eCommerce businesses:
• Have rigorous data quality management processes to clean, validate, and standardize incoming data. It will prevent errors and ensure decisions are based on reliable information.
• Retail analytics incorporates data from multiple sources. Integrating customer data from transaction records, service interactions, etc., can provide a detailed view of the customer journey and help uncover deeper insights for informed decision-making.
• Implement real-time analytics to give eCommerce businesses a competitive edge by facilitating immediate responses to customer behavior and market conditions, such as launching targeted marketing campaigns and adjusting prices.
• Use analytical tools and technologies like predictive analytics, ML, and AI to enhance insights obtained from data. These tools can identify patterns and predict trends missed by traditional analysis methods.
• Regularly review and update analytics strategies to adapt to new market conditions and technologies. Continuous learning from past analytics results would help in optimizing future tactics.
Summary
Retail analytics is vital in the eCommerce retail business, leveraging advanced technologies like AI and ML to drive growth and fulfill consumer demands. It allows businesses to understand customer behaviour, optimize inventory, enhance marketing efforts, and create targeted discount offers. It uses detailed data analysis from various sources, such as POS devices, customer interactions, and inventory systems. It also aids in making informed decisions, from customer engagement to designing pricing strategies, ensuring businesses stay competitive in the digital market.
How can Tx help with Retail Data Analytics?
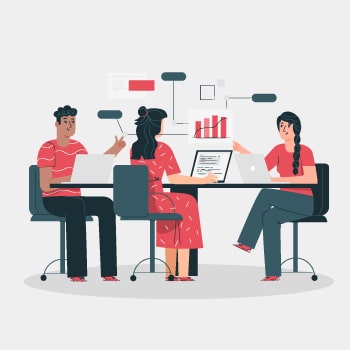
At Tx, we understand that the complex eCommerce retail architect must be more dynamic and data-driven in the current digital environment. Our expertise in next-gen IT solutions like RPA, AI, and ML enables businesses to solve the complexities of their modern data infrastructure. Partnering with Tx will give you the following benefits:
• Customized testing approach to ensure data quality at various phases of the ETL processes. We have partnered with QuerySurge to automate the Extract, Transform, and Load verification process.
• Thorough data sanity checks to verify data integrity, ensuring your analytics are based on reliable and precise information.
• Implement data visualization techniques to clarify complex insights, making it easier for businesses to make informed decisions quickly.
• Strong emphasis on compliance and security protocols during the data analytics process.
• Enable modernization of legacy data systems to ensure your analytics infrastructure can handle current data demands efficiently.
• Ability to monitor key performance metrics and adapt to evolving market conditions and consumer trends.
To know more, contact our experts now.