Recommended Blogs
Generative AI In Insurance | Automate Risk Assessment and Claim Processing
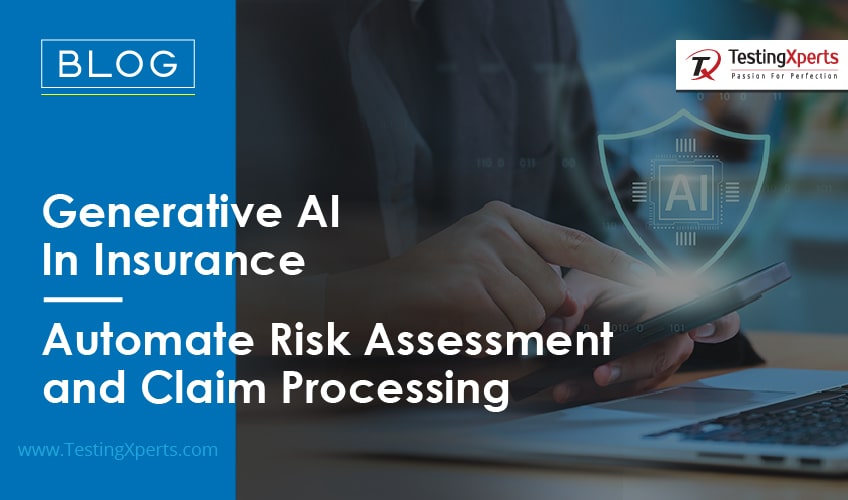
The rising demand for more efficient and precise risk evaluation and claim handling approaches is making the integration of generative Artificial Intelligence crucial. It is not just a visionary thought but a present reality, as data from late years demonstrates an enormous move towards AI-powered technologies. It is estimated that by 2030, AI utilization in the insurance sector will achieve up to $300 billion. This pattern is anticipated to continue, as AI can diminish the time expected to deal with a claim by 30% to 50%, and practically 96% of senior insurance administrators are putting resources into AI innovations to streamline expenses.
While AI offers numerous opportunities to increase efficiency within the insurance sector, its role in comprehending and pricing risks associated with climate change proves increasingly important. Changing weather patterns create catastrophic risks that are challenging to evaluate and incorporate into pricing models. AI can also support new product lines and claims pricing, especially concerning cyber risks that exceed the capabilities of traditional methods. This technology additionally addresses burnout faced by underwriting departments by automating routine and tedious jobs. Integrating AI within insurance has become essential not just to remain competitive but for the industry’s sustainability and evolution over time. Capitalizing on AI’s benefits to navigate complex risk and claims scenarios promises to provide enhanced accuracy, efficiency, and customer satisfaction. Ultimately, the future of insurance relies upon utilizing this technology to its fullest potential.
Role of Gen AI in the Insurance Industry
The insurers’ approach towards risk appraisal and processing claims is altering by including generative AI in the insurance industry. The move towards more AI-powered solutions or the inclusion of innovative technologies in any existing business is driven by the urge to raise accuracy, speed, and cost-saving regarding insurance processes.
Improved Risk Assessment:
Generative AI uses sophisticated algorithms and advanced machine learning to analyze massive data sets, including historically reported claims, environmental information, and customer behavior. Through such extensive coverage, generative analytics can predict potential risks very accurately. For instance, AI may recognize a pattern that shows more possibility of claims in some regions or for some circumstances and help insurers change the risk models.
Efficient Claims Handling:
By utilizing artificial intelligence for tasks like data input, fraud identification, and preliminary claim evaluation, the claims process is expedited while also decreasing human mistakes. This allows employees to focus their efforts on more intricate tasks. Moreover, AI can detect irregularities within claims information that could point to deception, helping to safeguard the company from monetary losses.
Custom-Built Insurance Plans:
Through artificial intelligence, insurers can draft individualized insurance proposals by examining policyholder information. These proposals are founded on singular risk profiles and can comprise unique premium prices and insurance alternatives. This tailored approach guarantees clients obtain insurance arrangements matched to their exact demands and hazard susceptibilities.
Cost Efficiency Through Technological Advancement:
The automation and effectiveness created by artificial intelligence result in considerable cost reduction for insurance providers. Savings originate from lessened labor requirements, decreased mistakes, and more productive use of resources. Furthermore, by enhancing the precision of risk evaluation, insurers can price their products more appropriately, avoiding overcommitment to high-risk situations.
Insurers can offer personalized services by leveraging artificial intelligence. They can create custom-tailored insurance plans that match customers’ risk profiles and preferences. This personalization also applies to proactive communication. Artificial intelligence systems can send individualized updates and advice based on each customer’s unique situation. Additionally, AI-powered tools like chatbots and virtual assistants provide customers with instant, round-the-clock access to information and support. These tools can handle routine inquiries, policy updates, and even initial claim registrations. As a result, customers receive quicker responses and higher satisfaction levels. The integration of artificial intelligence streamlines operational efficiency. It significantly enhances engagement and satisfaction levels across the insurance sector.
Transforming Risk Assessment with Generative AI
With its advanced algorithms and machine learning capabilities, Gen-AI enables insurers to analyze risk with unprecedented accuracy and depth. By processing vast and complex datasets, AI provides previously unattainable insights, leading to more informed and precise risk evaluation.
Advanced Data Analysis:
Gen-AI delves deep into vast amounts of data, including structured and unstructured data. This encompasses not just the basic demographic and historical data of policyholders but also broader socio-economic data, environmental factors, and even real-time data streams. By analyzing these diverse data sets, AI uncovers subtle correlations and patterns that traditional methods might overlook, leading to a richer, multi-dimensional understanding of risk factors.
Predictive Modeling:
AI’s predictive modeling uses complex algorithms to forecast future claims and risk scenarios. This forward-looking approach is especially crucial in areas with emerging risks, like cyber insurance, where historical data may be limited or non-representative. Predictive modelling allows insurers to proactively adjust their risk strategies, pricing, and underwriting criteria per anticipated future trends and events.
Customized Risk Assessment:
AI enables a shift from generalized risk assessment models to individualized evaluations. This personalization extends to analyzing each policyholder’s behavioral patterns, lifestyle choices, and unique personal circumstances. Such detailed analysis allows for the creation of insurance products and pricing models that accurately reflect the individual risk profile, leading to more impartial insurance premiums and coverage.
Fraud Detection:
AI’s role in fraud detection is multifaceted. It includes analyzing claims for unusual patterns, cross-referencing claims against known fraud indicators, and scanning social media and other public data sources for discrepancies. This comprehensive approach to fraud detection minimizes financial losses due to fraudulent claims and deters potential fraudsters, thus maintaining the overall integrity of the insurance process.
Real-time Risk Monitoring:
The dynamic nature of risks today, especially with the impact of climate change and rapid socio-economic shifts, requires real-time risk monitoring. AI systems can constantly analyze data streams – from weather patterns to economic indicators – providing insurers with up-to-the-minute insights. This real-time analysis is critical for dynamically adjusting risk models and responding promptly to emerging risk factors.
Regulatory Compliance:
In an industry heavily regulated and subject to constant legal changes, AI’s ability to ensure compliance is invaluable. AI systems can be trained to understand and monitor compliance requirements across jurisdictions. They can automatically adjust risk assessment models and insurance products to align with the latest regulations, safeguarding insurance companies against legal risks and penalties.
Claim Processing Through Gen-AI
Incorporating gen-AI into claim processing is revolutionizing the insurance industry, significantly improving efficiency, accuracy, and customer satisfaction. This advanced technology transforms the traditional methods of handling insurance claims, introducing a level of automation and intelligence that was previously unattainable.
Automation of Claims Handling:
Generative AI introduces a high degree of automation in routine tasks such as data entry, initial claim documentation, and basic assessment. This technology can quickly organize large amounts of claim data, extract relevant information, and perform initial evaluations based on pre-set criteria. This level of automation reduces the processing time for each claim, minimizes human errors, and allows insurance professionals to concentrate on more complex, judgment-based aspects of claims handling.
Enhanced Fraud Detection:
AI’s capability in fraud detection involves sophisticated pattern recognition and anomaly detection algorithms. These systems are trained on vast datasets of historical claims, enabling them to identify irregularities and suspicious patterns that might indicate fraudulent activities. For instance, an AI system can flag claims with atypical patterns in billing, uncommon treatment protocols for specific injuries, or inconsistencies in claim narratives. This proactive approach to detecting fraud helps mitigate financial losses and maintain the credibility of the insurance process.
Faster Claim Settlements:
AI accelerates the claim settlement process by rapidly processing and evaluating claims. Advanced algorithms can assess the validity of a claim, estimate the appropriate payout amount, and even automate the payment process in clear-cut cases. This speedy processing leads to quicker settlements, significantly reducing the waiting period for customers. A faster settlement process boosts operational efficiency and significantly enhances customer satisfaction and trust in the insurance provider.
Improved Accuracy and Consistency:
By leveraging AI, insurers ensure that every claim is processed accurately and uniformly. AI systems follow precise algorithms and criteria, eliminating the subjectivity and inconsistency that can arise with manual processing. This uniformity ensures that similar claims are treated alike, providing a fair and predictable claim-handling experience for policyholders.
Data-Driven Decision Making:
AI enables insurers to make decisions based on comprehensive data analysis. It can identify claim types, frequency, and severity trends, providing valuable insights into risk management and policy adjustments. For instance, if AI analysis reveals an increase in a particular type of claim in a specific geographic area, insurers can adjust their underwriting criteria or risk mitigation strategies accordingly.
Personalized Customer Interaction:
AI-powered tools like chatbots provide personalized, real-time customer interaction. They can update customers on claim status, answer FAQs, and even guide them through the claim filing process. This level of personalized service makes the claim process more engaging and less stressful for customers. Furthermore, AI systems can adapt their communication style to match customer preferences, creating a more empathetic and human-like interaction.
Integrating Generative AI into Existing Insurance Models
Integrating generative AI into existing insurance models redefines how insurance companies operate, assess risk, process claims, and interact with customers. The shift involves technical implementation and a strategic realignment of business models to leverage AI’s capabilities fully.
Strategic Planning and Implementation:
Insurance companies must thoroughly analyze key areas AI can significantly impact, such as automating claims processing or enhancing risk assessment models. A comprehensive plan should be laid out, detailing the integration timeline, required technological upgrades, and potential impact on current workflows. This plan should also address how employees interact with the new AI systems and the expected changes in their roles.
Data Infrastructure and Management:
Effective AI integration relies on a strong data infrastructure. Insurance companies must evaluate and potentially upgrade their data storage, processing, and security systems. This involves ensuring the capability to handle large volumes of varied data and maintaining data integrity and security. Proper data management is key to training AI models effectively and ensuring they operate correctly and reliably.
Regulatory Compliance and Ethical Considerations:
As AI models are integrated, it’s crucial to stay compliant with existing laws and regulations, especially data privacy and consumer protection. Additionally, companies must consider ethical aspects, such as ensuring AI algorithms are free from biases and maintaining transparency in AI-driven decisions. Ethical guidelines for AI use are essential to maintain public trust and avoid legal issues.
Workforce Training and Adaptation:
The integration of AI will transform traditional roles in the insurance industry. Employees need to be trained to understand and effectively collaborate with AI systems. This training should focus on how AI can augment human decision-making and improve efficiency. Addressing concerns or misconceptions about AI in the workforce is essential to ensure a smooth transition.
Continuous Monitoring and Evolution:
AI systems aren’t static. They require ongoing monitoring, updating, and refinement. Insurance companies must establish procedures for regularly evaluating AI performance, ensuring that the models remain accurate and effective in changing market conditions. This continuous evolution is crucial to maintaining AI systems’ relevance and efficacy in the dynamic insurance landscape.
Customer Engagement and Transparency:
When integrating AI into customer service, transparency is key. Customers should be informed about how AI is used, mainly how their data is processed and the benefits they gain from AI integration. Clear communication helps build trust and reassures customers that AI is being used to enhance their service experience.
Conclusion
Integrating generative AI into the insurance industry marks a fundamental transformation, indicating improved efficiency, accuracy, and customer-centric services. This technological advancement represents a shift in how insurance companies operate, assess risks, process claims, and engage with customers. The continuous evolution and strategic implementation of AI will be vital in handling the insurance sector’s complex structure. Implementing generative AI is necessary for insurers aiming to stay relevant and provide exceptional service in an increasingly digital world.
How Can TestingXperts Help with Generative AI Testing?
TestingXperts provides tailored testing services to guarantee robust and effective testing of generative AI solutions. Our specialists have experience dealing with the complexities of AI algorithms, confirming that they work as designed and delivering precise, dependable results. With a deep comprehension of AI’s technical and practical aspects, our experts provide extensive testing solutions that meet the requirements s of organizations utilizing generative AI.
Our AI testing specialists ensure that your AI systems achieve superior quality and productivity benchmarks through rigorous analysis and testing.
Recognizing that each AI application is unique, we offer customized testing strategies tailored to the specific requirements of each project. Our approach is not one-size-fits-all. We adapt our testing methods to suit each AI model’s unique challenges and objectives.
With AI applications handling sensitive data, security and compliance are paramount. TestingXperts strongly emphasizes testing for security vulnerabilities and ensuring compliance with all relevant regulations and standards.
We employ the latest tools and in-house accelerators, such as Tx-SmarTest, Tx-HyperAutomate, etc., in AI testing, enabling us to uncover issues and efficiently ensure AI systems’ robustness. Our toolkit includes advanced simulation environments, automated testing frameworks, and bespoke tools developed in-house.
At TestingXperts, we work closely with our clients throughout the testing process. This collaborative approach ensures that our testing strategies align with the client’s goals and that any issues are promptly addressed and resolved.
To know more, contact our QA experts now.
Discover more
Stay Updated
Subscribe for more info