Recommended Blogs
Implementing Data Governance in the Age Of Generative AI
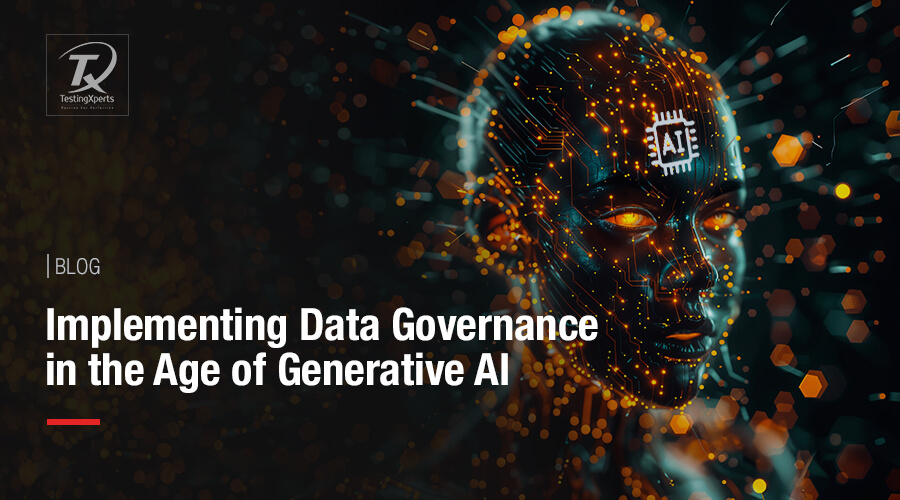
- Role of Data Governance in Generative AI
- How does GenAI Support Data Governance?
- Ensuring Better Data Governance When Implementing Gen AI Models?
- Why Partner with Tx for Data Governance?
- Summary
The generative AI era offers new opportunities to every individual, industry, and business. At the same time, the complexities and number of cyberattacks, regulations updates, expanding data estate, and demand for better data insights are all converging. This pressures business leaders to implement modern data governance practices and security strategies to ensure their AI readiness. Every digital touchpoint is a goldmine of data for any modern enterprise as it stands as the cornerstone for its development. However, if they truly want to benefit from this abundance of data in training their GenAI models, they must ensure its quality, manageability, security, and accessibility. And this makes it necessary for robust data governance in the generative AI era.
Gartner says more than 80% of organizations will use GenAI models or APIs by 2026. This technology has plenty of use cases in various industry verticals, such as:
• Marketing
• Sales
• Customer Service
• IT and Cybersecurity
• Research and Development
• Finance
• Supply Chain Management
• Product Development
• Manufacturing
Most use cases depend upon data analytics, where GenAI assists in improving data preprocessing and augmentation. It generates quality data for training models, improves data visualization, and automates analytics tasks.
Role of Data Governance in Generative AI

Data is a differentiator in a generative AI project, and a successful GenAI implementation depends on a feasible data strategy consisting of a robust data governance method. When an enterprise starts working with LLMs’ use cases, it must implement privacy and quality controls for responsible AI. However, the data generated from isolated systems or sources combined with a lack of data integration strategy results in data provisioning challenges for GenAI applications. Enterprises need an E2E strategy for data governance and management at every step of GenAI implementation, consisting of the following:
• Data ingestion
• Storing the data
• Querying the data
This would allow swiftly analyzing, visualizing, and running AI and ML models. With its policies, controls, and procedures, data governance ensures data security, quality, and integrity throughout its lifecycle. Data governance in generative AI implementation is essential for the following reasons:
• Establishing guidelines and ethical considerations to ensure the right use of data would help organizations swiftly handle GenAI complexities, such as avoiding biases, responsibly generating synthetic data, and preventing discriminatory results.
• Ensuring compliance with data protection laws and regulations like CCPA, GDPR, PCI DSS, etc.
• Preventing unauthorized access to confidential data by managing and implementing access controls, 2FAs, and other measures would mitigate the risk of GenAI app misuse and data/security breaches.
How does GenAI Support Data Governance?
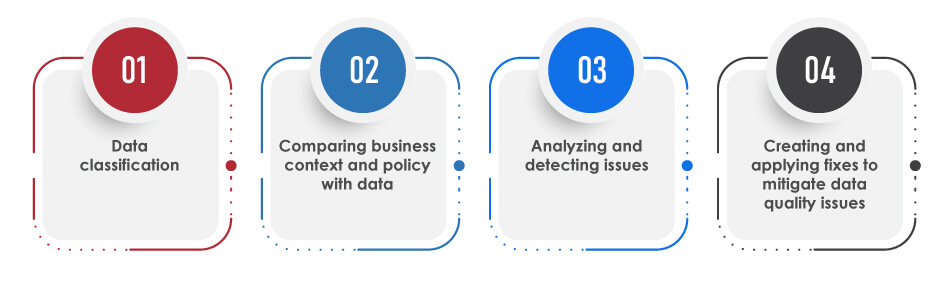
Generative AI automates data labelling, annotation, discovery, and profiling processes, offering a contextual data quality framework supported by distributed processing methods for scaling and speeding. It also creates a feedback loop for teams to optimize the data ecosystem and delivers advanced analytical insights to data scientists and business leaders.
Enterprises can address real-time challenges associated with siloed data by leveraging GenAI to define adaptive data governance parameters. This would add lineage tracking and adaptive capabilities to ensure the accuracy of datasets. The adaptive data governance powered by GenAI utilizes AI and ML algorithms to identify critical data across structured and unstructured sources. The traditional AI/ML focused on automating and scaling various data governance processes, which include:
• Data classification.
• Comparing business context and policy with data.
• Analyzing and detecting issues.
• Creating and applying fixes to mitigate data quality issues.
Generative AI has the potential to further enhance and accelerate these capabilities in data governance processes. It can:
• Categorize and add metadata tags to unstructured data depending on content type/themes.
• Develop synthetic data for training, developing, and testing models.
• Draft detailed reporting or dataset lineage to enhance trust.
• Manage data access control based on roles, usage, policies, and permissions.
• Utilizing regulatory intelligence context from policy documents as technical controls.
Ensuring Better Data Governance When Implementing Gen AI Models?
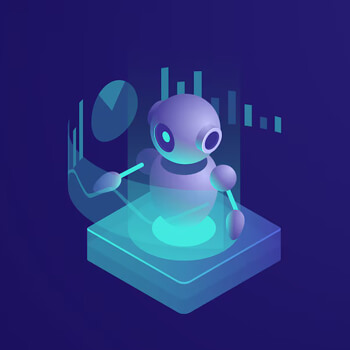
Many business leaders have second thoughts about implementing generative AI models into their data analytics functions due to challenges such as data leaks, unstructured data management, biased results, etc. However, having scalable and compatible data governance practices and technology would allow them to utilize GenAI models’ full potential and meet their organizational goals. Now the question is, “How can enterprises combine both in the most effective way possible?”
Organizations can start by implementing a comprehensive data governance strategy covering quality and privacy parameters regarding responsible AI. They can also work with LLMs for data analytics purposes. Unsurprisingly, a large part of enterprise data comes from siloed and unstructured sources, raising privacy and accuracy concerns. Businesses can mitigate such challenges by adopting E2E data governance and management policies. Utilizing data governance practices for generative AI implementation would give several benefits, such as:
Improved data accuracy while ensuring its completeness, consistency, and uniqueness.
Ensuring data protection from unauthorized/malicious access, thus reducing data breaching risks and remaining compliant with regulatory/legal standards.
Enabling connection between data across different applications, sources, and platforms. This eliminates data silos and provides a comprehensive view of data.
Allowing businesses to identify, understand, and utilize data efficiently and effectively. It would increase awareness about data governance for generative AI implementation and promote a data-driven approach within the enterprise.
Why Partner with Tx for Data Governance in Generative AI Implementation?
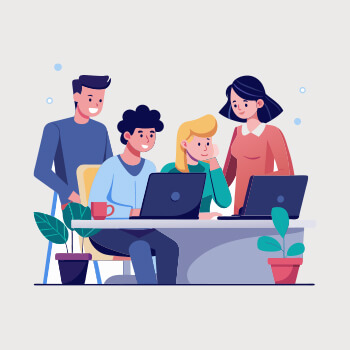
We at Tx have years of experience documenting and streamlining business processes. Our AI and security experts have hands-on experience in multiple business verticals. We can assist you in devising a thorough data governance strategy to facilitate your GenAI implementation plan. Our data governance approach covers the following:
• We define a data governance strategy, document goals, select a data model, and secure client approval.
• Defining and developing roles, policies, and procedures for data management, establishing a data quality team, documenting policies with governance working group approval, and communicating them to stakeholders, highlighting their business impact.
• Data compliance is ensured by adhering to laws such as CCPA and GDPR.
• Ensure your data is secured and accessible to the authorized person, and have information about your data assets with actionable insights.
• Ensuring your generative AI project is secure and reliable by utilizing our in-house accelerators (Tx-Secure and Tx-Insights).
Summary
Generative AI presents new opportunities but requires strong data governance to handle challenges like cyberattacks, regulations, and data quality. Effective data governance ensures data integrity, security, and compliance with laws such as GDPR and CCPA. Generative AI enhances governance by automating tasks like data labeling and profiling, improving management, and supporting adaptive governance. A comprehensive data strategy is crucial for successful GenAI implementation, addressing privacy, access control, and data integration. Tx offers expertise in developing and managing robust data governance strategies to support effective and secure GenAI projects.
To know more, contact our experts now.
Discover more
Stay Updated
Subscribe for more info