Recommended Blogs
AI in Wealth Management: Use Cases and Benefits
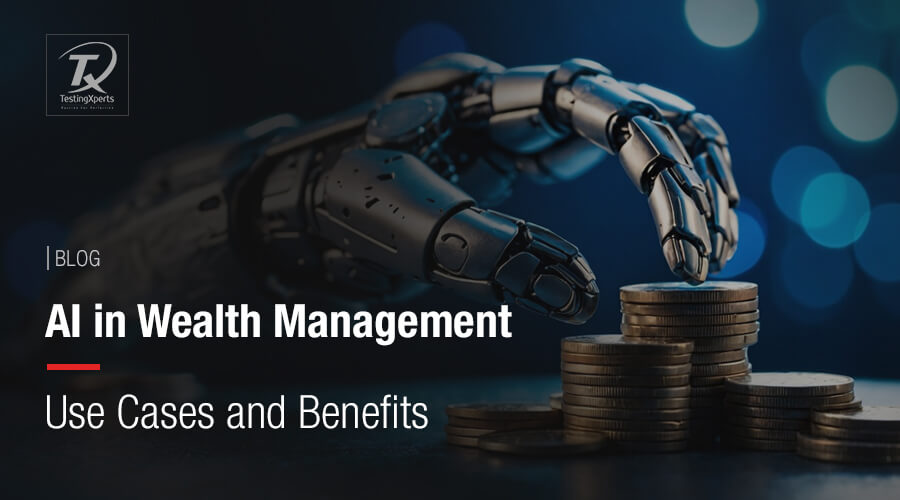
- Significance of AI in Wealth Management
- Traditional vs AI-based Wealth Management
- 5 Use Cases of AI in Wealth Management
- Benefits of Using AI in Wealth Management
- How Can Tx Assist in Implementing AI for Wealth Management?
- Summary
Artificial intelligence (AI) is becoming a core aspect of many industries, including financial services. Although some clients still prefer a human advisor, working with AI for wealth management has great potential. According to a survey, almost 60% of financial advisors are interested in using ChatGPT. Organizations focusing on growth must understand how and where AI technology will fit into their business plan. Let’s take a quick look at some facts about financial advisor (FAs) and their relationship with AI:
- 83% of FAs believe AI will directly and measurably impact the client-advisor relationship.
- 55% of FAs said that AI will either transform or revolutionize the future of financial advisory.
- 9 out of 10 FAs believe that AI has the potential to grow their business organically by more than 20%.
These statistics show the level of agreement among financial advisors and wealth executives. Integrating AI in wealth management is necessary to stay ahead of the curve. It offers a more efficient, data-driven, and personalized approach to wealth management.
Significance of AI in Wealth Management
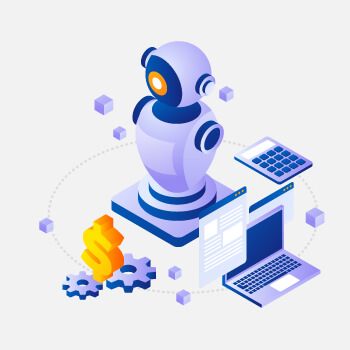
AI and ML solutions have transformed the fintech industry for over a decade. Users have seen improvements in various areas, from underwriting optimization to detecting fraud scores. This technology has great potential to transform various sectors, including commerce, education, gaming, and more, and could be a great asset in wealth management. According to a projection, the assets managed by AI-based advisors will save up to $5.9 trillion by 2027. Businesses will use AI technologies like predictive and genAI to offer financial advice, forecast investment performance, and map the latest market trends.
Predictive AI will provide projections based on trends or existing datasets. It will assist in identifying investment opportunities and effectively managing risks. This will also improve risk assessment to ensure financial businesses’ investment strategies sync with their client’s preferences and financial goals. Meanwhile, genAI will produce new data based on existing data. Another way is using machine learning to teach computers by identifying data patterns. Overall, AI in wealth management will assist businesses in making informed, data-driven investment decisions and swiftly target market trends. However, before integrating AI into wealth management, one must also consider performing AI testing to ensure seamless implementation.
Traditional vs AI-based Wealth Management
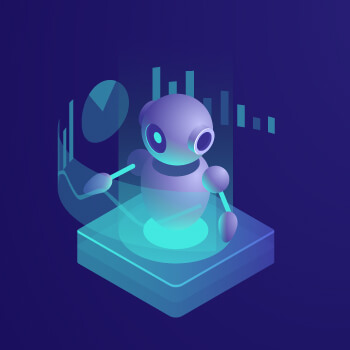
Aspect Traditional Wealth Management AI-based Wealth Management Decision-Making It relies on human expertise and intuition Driven by data analysis, algorithms, and predictive modeling Personalization Customization based on manual client interactions and assessments Highly personalized through continuous data-driven insights Speed of Analysis Slower due to manual data processing and review Faster with real-time data processing and automated decision-making Cost Typically, higher due to manual labor and expert fees Often lower as AI reduces the need for extensive human intervention Human Interaction High level of direct client interaction and communication Limited direct interaction; mostly automated with occasional human oversight Risk Management Depends on the experience and judgment of financial advisors Utilizes advanced algorithms to predict and mitigate risks Accessibility Generally accessible to clients with higher wealth More accessible to a broader range of clients due to lower costs Adaptability Less flexible, slower to adapt to market changes Quickly adapts to changing market conditions through continuous learning
5 Use Cases of AI in Wealth Management
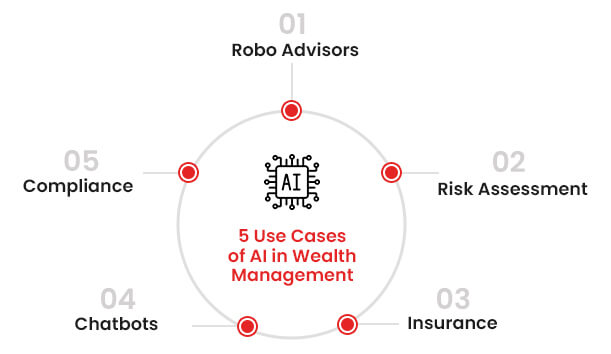
AI assists in improving customer interactions to optimize investment strategies. It leverages its unique abilities to improve the accuracy and efficiency of the wealth management process and open doors to unparalleled customization and client engagement. Let’s take a close look at some of the potential use cases of AI in wealth management:
Robo Advisors
These AI-based financial advisor apps offer clients automated and algorithm-driven investment strategies. Robo advisors leverage AI to assess client’s choices, investment goals, and risk tolerance to deliver personalized financial advice. These apps are cost-effective, highly accessible, and have a user-friendly UI, which makes wealth management more inclusive to diverse regions. With a data-driven approach and minimal human intervention, robo-advisors can upscale portfolio diversification and assist businesses in achieving their financial goals.
Risk Assessment
Businesses can optimize risk assessment for wealth management by leveraging data analytics and predictive algorithms. AI-driven tools can continuously monitor and detect the latest market trends. AI can also analyze clients’ financial profiles and risk assessment reports, allowing wealth managers to develop customized strategies. It improves the responsiveness of risk management in wealth management, ensuring financial security.
Insurance
In insurance, AI streamlines the assessment and processing of coverage. AI algorithms analyze clients’ data to select the relevant insurance products and coverage levels. They continuously monitor and adjust insurance portfolios to sync with changing financial goals and life circumstances. Lastly, AI in wealth management in the insurance industry improves long-term financial planning and investment risk protection.
Chatbots
AI-driven chatbots efficiently handle customer requests with their 24/7 virtual assistant. They respond promptly to account balances, appointment scheduling, and transaction history requests. Their NLP capabilities allow them to understand and respond to client queries like humans. This helps streamline wealth management operations and improves business-client relationships.
Compliance
Compliance management is one of the important use cases for AI in wealth management. AI-driven solutions assist businesses in streamlining the complex and dynamic landscape of regulatory standards. It is done by automating tasks such as AML screening, transaction handling, and performing KYC checks. It offers real-time compliance checks to allow wealth management businesses to maintain high customer security and trust. AI also helps in reducing human errors and operational costs.
Benefits of Leveraging AI in Wealth Management
Businesses can reap numerous benefits from leveraging AI technologies in wealth management. Some of them are given below:
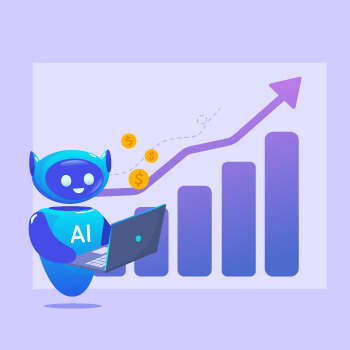
AI assists in customizing investment strategies based on business goals and requirements to ensure a personalized wealth management experience. This allows businesses to achieve their financial goals seamlessly with high-risk tolerance and long-term financial aspirations.
Businesses can leverage data-driven insights and recommendations to improve the quality of their wealth management decisions. This leads to informed and accurate choices, maximizing ROI and minimizing wealth management risks.
AI assists businesses in assessing and managing investment risks effectively. It protects and grows wealth by continuously analyzing market data and alerting about potential risks. Maintaining a secure and profitable financial ecosystem also minimizes the chances of financial losses.
Businesses can discover diverse investment opportunities across various classes. They can spread the investments across different assets so that the adverse impact on one class does not affect the potential gains from other classes. This leads to more balanced and resilient wealth management strategies.
AI automates repetitive admin tasks and helps wealth managers focus their time and resources on strategic aspects of portfolio management. It also helps optimize investment strategies and their outcomes.
AI-driven trading algorithms can execute trades at optimal times, maximizing returns while minimizing risks. These algorithms can process large datasets and make split-second decisions impossible for human traders.
AI can automatically rebalance portfolios based on changing market conditions or shifts in a client’s financial goals. This ensures that portfolios remain aligned with the client’s risk tolerance and investment objectives.
How Can Tx Assist in Implementing AI for Wealth Management?
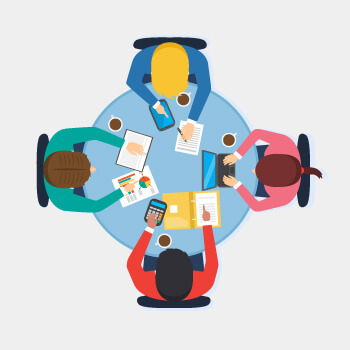
AI will play a crucial role in wealth management by improving transaction security, user trust, and transparency. Wealth management firms must integrate AI solutions seamlessly into their operations as they focus on enhancing CX and data analytics. Tx has in-depth knowledge of testing AI-based solutions and Fintech apps, covering a broad range of capital markets, complex order management systems, and banking applications. This would consistently ensure the best UX for your users. Our AI-based in-house accelerators, Tx-Automate, Tx-SmarTest, Tx-HyperAutomate, etc., can positively impact your time-to-market.
Summary
Integrating AI in wealth management is a transformative shift in the financial industry. Its diverse use case displays a wide range of applications of this technology. AI enables wealth management firms to provide high-quality services at cost-effective rates while enhancing risk management, CX, and customization. Need help with AI testing for wealth management? Contact our financial domain experts to learn more about the business benefits of testing AI-based solutions before integrating with wealth management services.
Discover more
Stay Updated
Subscribe for more info